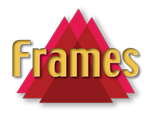
Resource Catalog
Document
Background: Fuel breaks aim to reduce the energetic progression of a wildfire, facilitating safe and efficient suppression. Changes in fire regimes are creating increasingly complex scenarios in which a higher percentage of wildfires exceed control capabilities, and a significant increase in firefighting costs is expected. Therefore, it is necessary to redefine fuel break networks incorporating science-based criteria. This change entails the improvement of the existing fuel breaks, the abandonment of those whose effectiveness does not justify the investment in their maintenance, and the development of new optimized designs. Fuel break effectiveness is understood as the probability of controlling a fire in the treated area. We analyzed 563 intersections between fires and fuel breaks that occurred during wildfires from 2011 to 2018 considering topographic, meteorological, fuel, design feature, suppression, and fire behavior factors. The main goal of this study is to quantitatively analyze the effectiveness of fuel breaks during wildfires in southern Spain and to develop models to predict potential fuel break effectiveness in fire containment capabilities by comparing machine learning techniques with a classic statistical approach.
Results: Fuel breaks were effective in containing the fire in 46.9% of cases. The most influential factors in effectiveness were the type of suppression work executed on fuel breaks (aerial, ground, or combined firefighting), the flame length, and the intersection angle between the fire and fuel break. Although the most accurate results were achieved with an artificial neural network, a decision tree could be the easiest model for end-user operational application.
Conclusions: This study entails a change in effectiveness assessment to an empirical approach in real wildfires in Spain. Our findings can be used to support decision-making for optimizing fire containment capability and firefighter safety.
Cataloging Information
- ANN - artificial neural networks
- decision tree
- fire containment capability
- fire suppression
- firebreak
- firefighter safety
- logistic regression
- preparedness
- quantitative analysis
- Spain